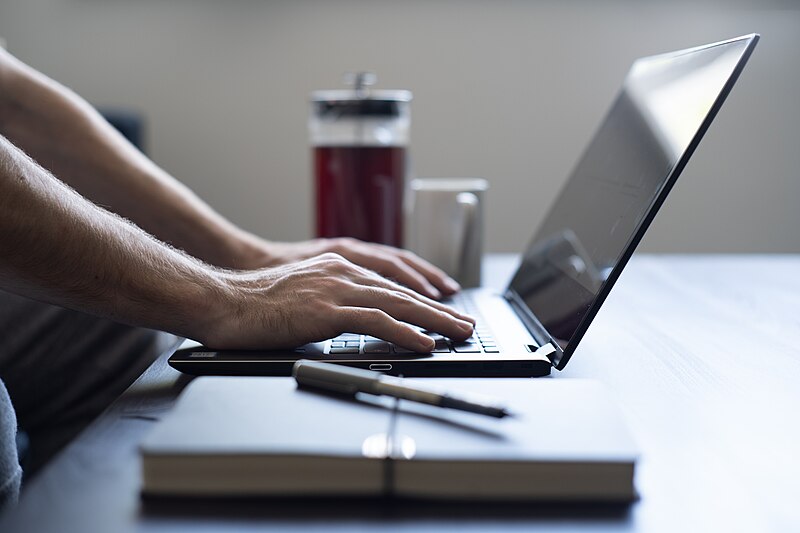
In the gig economy, the customer isn’t king. They’re the emperor.
Workers on Uber, Lyft, TaskRabbit, Instacart, Fiverr, Upwork, and other labor platforms are at the mercy of algorithms that funnel jobs their way based on customer ratings. The better ratings, the better the assignments. By using customers for control, the platforms have ditched traditional management and gained tremendous efficiencies for themselves and for their customers. Who doesn’t love the ease of calling a rideshare to their exact location with a few taps on a cellphone, compared with standing on a street corner to hail a cab?
These platforms may have put customers on a throne, but gig workers aren’t simply content to be ruled. They are finding covert ways to take back some measure of control. In our study, we uncovered several different tactics that gig workers employ to push back against their digital bosses — and they adjust those tactics depending on the stage of the work. They have the most latitude for resistance at the beginning of the work process, less in the middle, and very little after the work has concluded.
Before we highlight our findings, we need to recognize that labor platforms have made the customer service interaction longer. This elongation is important because it affects how workers resist. Let’s use a traditional taxi ride as an example. The rider signals, the cabbie decides if they want to stop, and the ride ends with both parties unlikely to interact again. If the customer is displeased, they can withhold a tip or call the taxi agency to complain. The customer’s feedback isn’t shared with the next rider, and the whole affair is a brief transaction.
But in the gig economy, the customer’s journey is more complicated. The driver may choose to accept an incoming ride request or not, basing their decision on information displayed in the app, including the customer’s name and pick-up location. After the ride is over, the customer uses the app to rate the driver, which adds data to the algorithmic management system that matches the driver to future assignments. The transaction is longer for the customer, and the outcome more durable for the driver.
For our study, we examined both a ride-hailing platform and a freelance platform that paired customers with professionals in web development, graphic design, marketing, and similar disciplines. The ride-hail data came from participant observation, interviews, and social media content collected in a previous study done by one of the authors (who spent time as both a rider and driver to gather information). The freelance platform provided access to 200 anonymized communications records related to project work as well as online forums. The average project lasted three months.
In our analysis, we found three distinct worker resistance tactics described below and summarized in Figure 1 below:
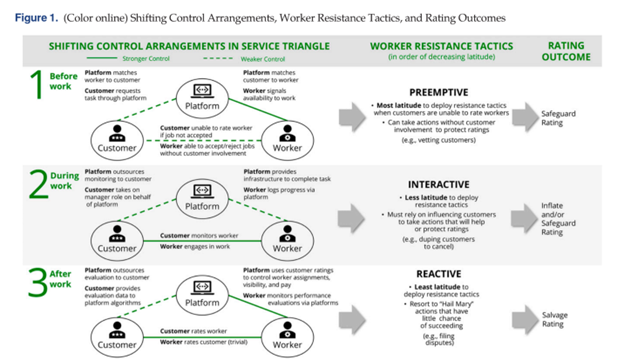
Stage 1
Gig workers have the most latitude to take action and resist before the work begins. In this stage, they use preemptive tactics with the goal of ensuring a good rating. Most commonly, they vet the customer, looking for indications that the person may be difficult to work with or rate them poorly.
Although both platforms prohibit rating discussions before work is completed, freelance workers often ask customers for a high rating as a condition of acceptance, or they offer a low bid on condition of a perfect rating. In ride-hailing, drivers call the rider first, ostensibly to verify their name and destination, but really to ascertain their personality. If the customer seems rude or unpleasant over the phone, they are more likely to leave a low rating. While drivers can cancel the ride they are penalized by the algorithmic management system with fewer plumb assignments. So, they often finesse the customer into canceling, which doesn’t hurt their standing on the app.
Stage 2
Gig workers have less latitude for resistance once the task is underway, they devise interactive tactics to safeguard their rating. For freelancers, this is achieved through segmenting work. They divide a project into smaller pieces, each with individual ratings, to boost their score. They also may hold part of the work hostage, releasing the product only if the customer gives them a high rating. If they feel like a project is going south, freelancers also may end the work early and refund part or all of the fee, with the request that the customer give them a good rating in exchange. Drivers can cancel bad rides while they are in progress, which prevents the customer from rating them.
All of these actions are risky, of course. When drivers cancel, they don’t get paid the full fare and increases their liability risk. When freelances cancel they are flouting the platform’s policy and could be kicked off the site. But many gig workers report that the risk is worth it to preserve their perfect rating and keep work coming their way.
Stage 3
In this final stage of the transaction, workers resort to reactive tactics to wrest control. Drivers who suspect a low rating from a rider will give the customer a low rating first, hoping the algorithm cancels out both ratings. Both drivers and freelancers who feel they’ve been rated unfairly can file a dispute with the platform. But they told us they feel like this is a “Hail Mary” attempt that will be unsuccessful because the platforms give more weight to customers’ ratings and feedback.
Customer Accountability
While labor platforms arguably expose gig workers to more jobs than they could obtain through word of mouth alone, digital bosses leave them with few outlets for resistance. The platforms have granted near total oversight to customers with little accountability for nefarious behavior like verbal abuse, sexual harassment, or theft. And gig workers don’t have a direct supervisor, union representative, or human resources administrator to help them fight back. They are beholden to the algorithmic management system. As one freelancer said, “your rating is everything.” Another said it was even “more important than money,” because high ratings help them get the next job.
Our research sheds light on how emerging technologies affect the scope of resistance among gig workers, who comprise a growing segment of the economy. While we don’t believe that businesses should abandon the “customer is king” axiom, we do think the unique challenges faced by gig workers perhaps require more consideration from the platforms — and from the customers. Think about the person who is sitting in traffic or waiting in line or cleaning your house or doing all the invisible work that it takes to make a dollar. Be patient, be flexible, tip well, and remember that with the click of a button, you have power over their livelihoods.
Authors
Lindsey Cameron is an Assistant Professor of Management and Sociology (by courtesy) at the Wharton School, University of Pennsylvania. She is also a fellow (member) at the Institute for Advanced Study, and an affiliated faculty at Harvard University’s Berkman Klein Center for Internet and Society, and at Data and Society Research Institute. Her research interests include algorithmic management, the gig economy and the future of work. Her research has been published in Administrative Science Quarterly, Organization Science, Journal of Applied Psychology, and Organizational and Human Decision Processes, among others, and received numerous awards. In 2023, she was named as one of the best 40 business school professors under 40 by Poets & Quants.
Hatim Rahman is an Assistant Professor of Management and Organizations at the Kellog School of Management, Northwestern University. His research investigates how artificial intelligence, undergirded by algorithms, is impacting work and employment relationships in organizations and labor markets. His research and teaching have received numerous awards, including the National Science Foundation CAREER award. In 2023, he was named as one of the best 40 business school professors under 40 by Poets & Quants.
Read More
Cameron, L. D., & Rahman, H. (2022). Expanding the locus of resistance: Understanding the co-constitution of control and resistance in the gig economy. Organization Science, 33(1), 38-58. https://doi.org/10.1287/orsc.2021.1557
Image: Microbizmag via Wikimedia Commons (CC by 2.0)